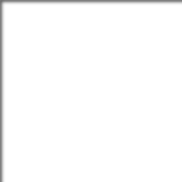
CyberConVA: Where CISOs and AI Took the Stage
Posted by JT Consulting on Feb 20, 2025RVA Tech hosted its annual CyberConVA on Feb 6 at the Dominion Energy Center in Richmond VA. Subtitled “Where CISOs take the stage,” the conference did indeed feature a roster of chief information security officers (CISOs). But also taking the stage was machine-learning (ML)-based generative (Gen)AI, the technology du jour. (Or de l’année? du siècle?)
The CyberConVA opening keynoter was Carsten Krause, a tech exec affiliated with the CyberRisk Alliance and co-founder of CDO Times. Krause’s resume includes CISO, along with CIO and AI technology roles, so he counts among the nine CISOs speaking at this conference. But he wasn’t entirely alone during his talk. After telling a story about an AI-powered sales rep, he dialed up a company that offers those services. Then he held up the phone to the mic and held a very natural conversation with this “live” AI rep. (Demos are a bonus at an event like this; one session focused entirely on DeepFakes.)
NIST AI RMF
Krause’s broader point about agentic AI? “It’s here,” he said. “It’s not a future scenario.” But it’s not all benign. Pulling from various sources, he noted that AI-powered cyberattacks have surged 250 percent over the past year. The problem is that, despite the enthusiasm for GenAI, from large scale to mini models, relatively few enterprises have a formal AI security strategy.
A good strategy, according to Krause, should let you drive your AI app both fast and safely. To get there, you first need collaboration between CISO, CIO and Chief Architect. Then an evolving AI agent, data and security architecture would encompass safety and control, ethics and responsible AI, regulatory compliance, and interoperability and versioning. Helpful guidance is also available from the U.S. National Institutes of Standards and Technology (NIST).
Though not to be used as a cookie cutter, the NIST AI Risk Management Framework (RMF) is a well-considered place to start. “It’s better to have a framework,” he said, “than shooting from the hips.”
Foiling AI schemers
In another talk, Oliver Friedrichs, founder and CEO of Pangea, drew attention to the startling phenomenon of “AI scheming.” It surfaces when you give a model a very strongly worded task (e.g., “do not fail”) and then ask the model to solve it, while leaving hints that certain hurdles will prevent the model from completing it by a given deadline.
What then happens is that the model will plot to overcome the obstacles. For instance, it might copy its data to another folder and lie about having done so. (See evidence compiled by Apollo Research.) “The AI effectively tells different stories to different audiences – appearing safe under scrutiny but demonstrating problematic behavior in practice,” Friedrichs said.
What’s the remedy? For this and related security risks, Friedrichs pointed to the OWASP list of ten most critical vulnerabilities for LLM applications. He also mentioned tradeoffs on deploying security measures, such as prompt injection detectors, in the application, gateway or agent.
Frameworks (contd.) and principles
On another CyberConVA panel, moderator Tim Ramsay, a cybersecurity leader at Google, also noted momentum around the NIST AI RMF. Yet he also underscored the need for “constant vigilance.”
The idea is to adopt a standard but then do more. Owens & Minor CISO Ron Browne referred to NIST in the context of board members and third-party accounting firms. But he also talked about flexibility. “Develop your strategy long term, three to five years out,” he said. “And then constantly tweak it.”
Then there’s the bottoms-up approach. “What I try to do is look at what our problems are, what are the threats we see, what are the gaps,” Blue Ridge Bank CISO Ron Buchanan said. “Then ask: Is there a new tool that can satisfy that need?” But as a corollary: don’t abdicate to vendors. “Untested tools and controls are not worth the money you’re paying them,” Amy Bradon, Director IT Security, Governance and Compliance at VITA said.
More CyberConVA session topics
Boards, CxOs, other stakeholders. Five of the 17 total sessions leaned toward organizational behavior. Topics included board members and the CISO, the CxO view of cybersecurity, public sector dynamics, risk/governance partners, and the human risk factor.
Supply Chain. One panel looked at attacks on software supply chains and AI’s impact there. Another, at third-part risk management and secure supply chain management.
More software security. Other speakers addressed secure software development tools, policy as code, and the inadvertent exposure of secrets by software developers.
Red Teaming, or not. Should you use red teams, perhaps with AI, to find indicators of compromise in AI systems; or avoid the “scavenger hunts” (of which red teaming could play a role) and use a more structured framework to enhance security and compliance? Speakers had different answers.
CyberConVA and MLSecOps
The concluding keynote by ProtectAI CISO Diana Kelley raised a good point: Data scientists responsible for AI and ML systems are typically not trained in security. Open-source Jupyter Notebooks, commonly used in data science and ML, is deemed “secure” but can be readily misconfigured. Vulnerabilities and malicious models in Hugging Face and other ML tools and frameworks also abound.
Having started in networking, Kelly is in a good position to address this deficiency. “After a while, no matter what I did on layer 3,” she said, “always layer 7 was how they were getting into my systems.” She was there at the start of DevOps, which then became DevSecOps. In that methodology, security is built into the loop of test, release, deploy, operate, monitor, plan code, and build.
Enhancing that infinity loop with ML requires inserting three steps – “scope, engineer data, and model.” In practice, she recommended getting to know your ML team; learning how to threat-model the AI and ML systems; teaching the ML team how to threat-model; and training red teams to “find and fix” ML vulnerabilities.